Nava Labs is a new, philanthropically funded division within Nava PBC focused on prototyping policy and systems changes within government programs and advocating for their adoption. Currently, we’re exploring how generative artificial intelligence (AI) might help increase access to public benefits such as the Special Supplemental Nutrition Program for Women, Infants, and Children (WIC) and the Supplemental Nutrition Assistance Program (SNAP). However, we recognize that AI (of any type) is not the right solution to every problem. We've taken the following approach to rigorously and critically assess when AI is appropriate to address certain challenges in the public sector. Your team might use this checklist to help determine whether AI is appropriate for a given use case.
Phase 1: Define the problem
_____ Conduct thorough research to understand problems as well as the needs and behaviors of those affected by the problems. Engaging with end users and stakeholders – including beneficiaries, frontline support staff, policy teams, and others – can provide valuable insights and help define the problem. For each problem area you identify, set concrete, research-driven goals for what any solution, AI or otherwise, should achieve. Learn more in Nava's toolkit on how to engage users and iterate.
Phase 2: Determine whether AI capabilities are appropriate
_____ Consider alternative solutions to AI and their feasibility. In many cases, a non-AI approach is likely to be more effective, cost-efficient, and easier to adopt. The goal is to find the most appropriate solution, not the most technologically advanced one. If AI doesn't offer a significant advantage over simpler methods, it may be wise to explore other options.
_____ Compare the functions and capabilities your solution needs against AI's core strengths. This includes "traditional" AI, which is stronger with pattern recognition, prediction, classification, and recommendation, and generative AI, which is stronger with information retrieval, content generation, summarization, and translation.
Clearly describe why or why not a given AI solution seems more promising than non-AI solutions. While AI has remarkable capabilities, it's not a panacea for all business challenges. AI is not particularly suited for tasks that require deep understanding and common sense (unless it is explicitly captured in the AI model), creativity, innovation, ethical decision-making, diplomacy or social skills, or adaptability in highly unpredictable situations.
_____ Consider how well an AI solution could integrate with your current workflows. AI capabilities might fit your problem, but using AI comes with tradeoffs and risks. These risks include adding administrative burdens or contributing to unintended consequences on end users and program outcomes. Problems may arise if you cannot integrate the AI solution into existing workflows or if it is difficult to adopt.
Phase 3: Assess your data, infrastructure, and operational readiness
____ Assess whether you have capacity to engage diverse perspectives in your solution design and evaluation design.
____ Ensure you have high-quality, representative datasets available for AI implementation. It is crucial that you have access to a sufficient quantity of high-quality data to ensure the accuracy and reliability of AI outputs. This data should cover the range and scope of your desired solution.
Note that custom machine learning (ML) models require large, clean datasets for training. Solutions that use generative AI with retrieval augmented generation (RAG) need enough relevant context data to draw from. And any AI solution needs high-quality test data to evaluate how well the model performs.
____ Determine how your data will remain accurate, complete, and up-to-date. Poor quality data can lead to inaccurate predictions and decisions, undermining how effective the AI can be.
____ Ensure the privacy and security of the data is in compliance with relevant regulations such as GDPR or HIPAA. If a generative AI solution relies on third party models, understand where and how the data is being sent and processed. You may also consider options that keep data within secure boundaries, such as Anthropic's Claude 3 LLMs available in AWS GovCloud or Azure's OpenAI Service in Azure GovCloud.
____ Ensure there are processes for obtaining consent to use beneficiary data in AI tools. Transparency is crucial to building trust with the public around the implementation of AI tools in service delivery. Moreover, it’s important to be specific when communicating what AI is and isn't doing in a given implementation. The level of detail you should provide varies based on the context, and your communication should always use concise, plain language. Also, consider which non-AI processes to leverage if beneficiaries opt out of using the AI system.
____ Assess whether your technical infrastructure can support AI integration. Understand what infrastructure changes you require before testing, deploying, and monitoring AI models. This includes hardware and software considerations as AI capabilities scale. If you find that you lack the necessary infrastructure and staffing/expertise, we advise that you address these fundamental issues before proceeding with AI implementation.
____ Consider the readiness of the intended end user of the AI solution. For example, if your staff or volunteers will use the AI system, consider the training and support they will need to understand expectations and drive adoption of the solution. If beneficiaries will use the AI system, consider the level of testing and quality assurance you will need to perform to ensure consistent and reliable performance.
Phase 4: Evaluate risks and create mitigation plans
____ Identify biases in underlying datasets and plan strategies to minimize bias. For instance, if the training data is biased against certain demographic groups, the outputs of AI are likely to be biased against those groups. Define how you will minimize bias, such as using diverse training datasets, conducting fairness audits, and correcting for biases in the overall service. Plan to monitor, assess, and correct for biased output periodically, particularly when datasets change or AI models are updated.
____ Consider how AI tools will impact different groups’ access to benefits, especially vulnerable populations. For example, if an AI customer support system doesn't have human alternatives, those who need more support navigating the benefits application process could face additional barriers.
____ Make plans to test and evaluate AI tools for potential adverse impacts before and after launching. When doing so, you should consider technical, operational, experiential, and ethical risks. This could include monitoring for technical failures or data breaches, gathering user feedback, or evaluating outputs and outcomes for unintended consequences of AI decisions. Importantly, you should use human review and automated testing. For example, an AI system might mistakenly flag legitimate claims as fraudulent, leading to delays and frustration for users. In this case, it’s crucial that a human review and correct the mistake.
____ Determine what supports and fallbacks you will put in place to mitigate potential failures. This includes plans for a human to review AI outputs and plans to fall back on non-AI processes in the event of significant failures or adverse impacts.
____ Determine whether the benefits of AI outweigh the risks, or if the risks can be mitigated. If risks, like rights and safety concerns, outweigh the benefits and can't be mitigated, AI may not be suitable for your use case. If the potential benefits outweigh the risks and/or risks can be adequately mitigated, and there is no better non-AI alternative to meet your goals, AI may be appropriate.
Visit our website to learn more about how we’ve employed these considerations to identify, prototype, and test generative AI solutions for addressing the challenges of navigating and enrolling in public benefits. You can also watch our recordings of Demo Day 1 and Demo Day 2, where we describe our work in depth.
Written by
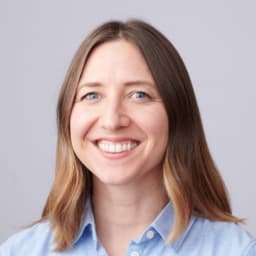
Partnerships and evaluations lead
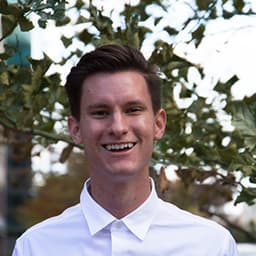
Software Engineer
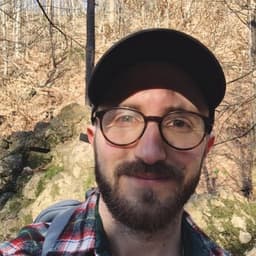
Designer/researcher
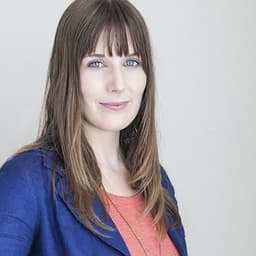
Senior Product Manager
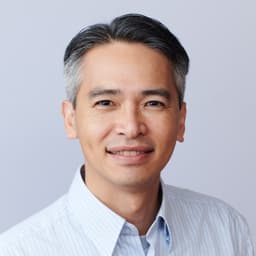
Principal engineer
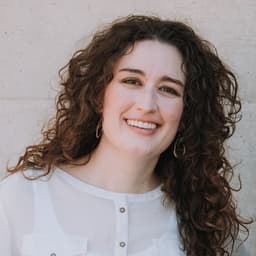
Partnerships and evaluation manager